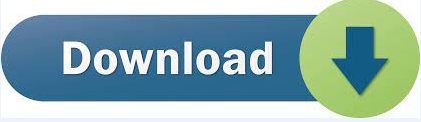
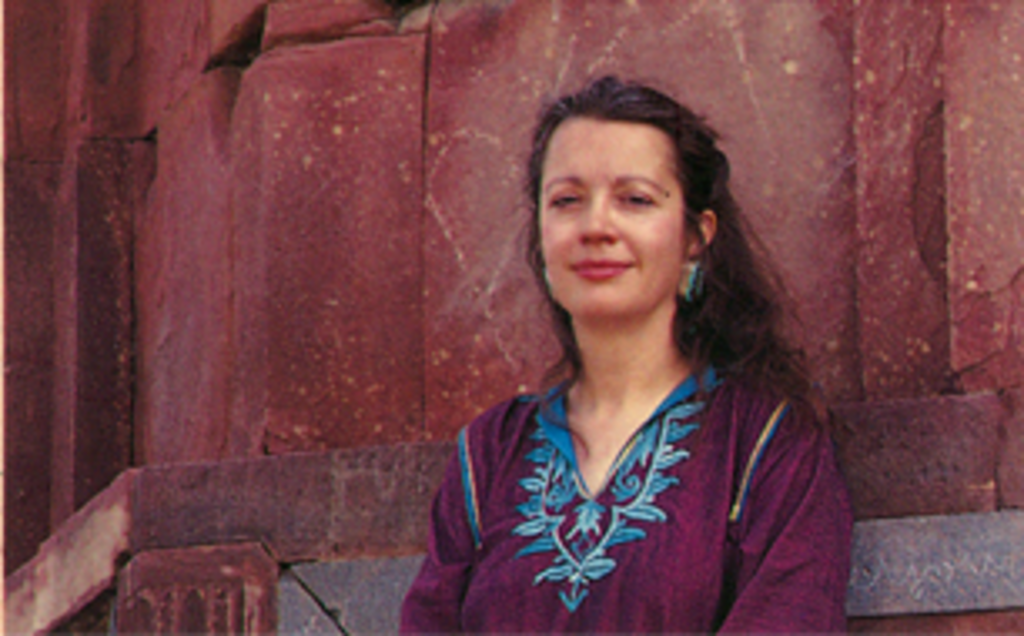
Defense Advanced Research Projects Agency (DARPA), nd Web 2 (2017). Explainable artificial intelligence (xai). In Proceedings of the 25th Conference on User Modeling, Adaptation and Personalization. Fine-Grained Open Learner Models: Complexity Versus Support.
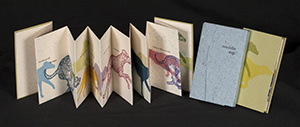
Digital and online symptom checkers and health assessment/triage services for urgent health problems: systematic review. Duncan Chambers, Anna J Cantrell, Maxine Johnson, Louise Preston, Susan K Baxter, Andrew Booth, and Janette Turner.The art of human-computer interface design(1990), 393–404. Conversation as direct manipulation: An iconoclastic view. Qualitative research in psychology 3, 2 (2006), 77–101. doctors, the ultimate test: a prospective study of patients presenting with abdominal pain. Andrew C Berry, Brooks D Cash, Madhuri S Mulekar, Bin Wang, Anne Melvin, and Bruce B Berry.Online symptom checker diagnostic and triage accuracy for HIV and hepatitis C. AC Berry, BD Cash, B Wang, MS Mulekar, AB Van Haneghan, K Yuquimpo, A Swaney, MC Marshall, and WK Green.ACM Transactions on Interactive Intelligent Systems (TiiS) 10, 2(2020), 1–37. Mental Models of Mere Mortals with Explanations of Reinforcement Learning. Andrew Anderson, Jonathan Dodge, Amrita Sadarangani, Zoe Juozapaitis, Evan Newman, Jed Irvine, Souti Chattopadhyay, Matthew Olson, Alan Fern, and Margaret Burnett.The use of artificially intelligent Self-Diagnosing digital platforms by the general public: Scoping review. Stephanie Aboueid, Rebecca H Liu, Binyam Negussie Desta, Ashok Chaurasia, and Shanil Ebrahim.We discuss how explanations are interwoven into conversation flow and present implications for future OSC designs. Our lab-controlled user study (N=20) found that explanations can significantly improve user experience in multiple aspects. Then, we designed a COVID-19 OSC that was enhanced with three types of explanations. We first conducted an interview study (N=25) to specify user needs for explanations from users of existing OSCs.

In this paper, we attempt to enhance diagnostic transparency by augmenting OSCs with explanations. However, intelligent systems’ lack of transparency and comprehensibility could lead to unintended consequences such as misleading users, especially in high-stakes areas such as healthcare. OSCs use algorithms such as machine learning to facilitate self-diagnosis and triage based on symptoms input by healthcare consumers. Online symptom checkers (OSC) are widely used intelligent systems in health contexts such as primary care, remote healthcare, and epidemic control.
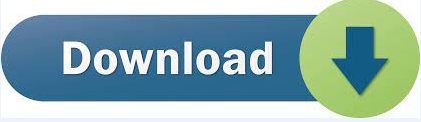